How AI and ML Enable Testing to Keep Up With IoT
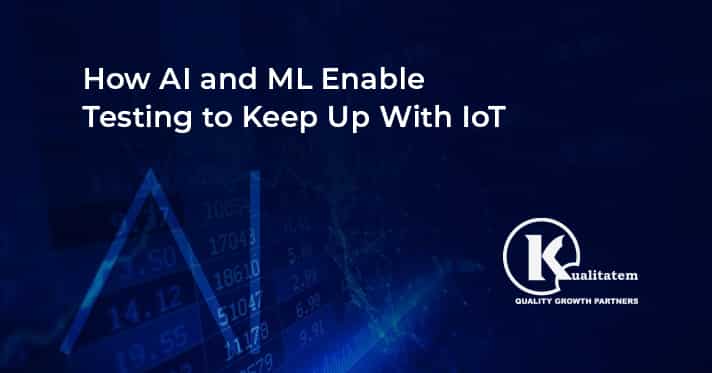
- January 22, 2020
- HibaSulaiman
According to recent statistics, the IoT market is expected to be valued at $1490.31 billion by 2024 owing to an increase in big and small IoT Testing Companies, leaving a fair gap between the demand and supply. Existing testing procedures and processes are not ready to face emerging challenges in the IoT world and are likely to result in delays in deployment, updates, and user-acceptance.
End-to-end software testing for IoT applications is even more complicated and it requires a well-planned strategy in place. Within IoT, companies are required to understand all the elements of an application, how they work together, how are they used by people, from the vast array of mobile phones and complex back ends to microservices managing a wide range of sensors and devices at the edge, as well as data collection, analytics, and decisions in the cloud. Needless to say, this is the end of manual testing; however, test automation also requires an overhaul to try and keep up.
This is where AI and machine learning has to step in. Automation of test execution is not sufficient as the entire testing process, from creation to analysis, needs to be automated. This requires intelligent models to auto-generate tests, with AI and ML allowing teams to analyze data from testing and to identify the bug patterns.
To continually test and monitor a system, models can be built automatically by AI-driven intelligent testing. Various AI approaches can be applied to network traffic, system resources, user interfaces, and so forth to build complete verification models. To achieve high levels of test coverage and confidence in complex IoT systems, expanding test automation beyond simple test execution and automating the creations of tests is the only way.
Upon auto-generation of tests, ML is then needed to direct testing towards areas that matter more – for instance, ‘learning’ the areas of an IoT system that are the riskiest and the area of most significance to the users and then focus on testing in those areas. Nowadays, it’s simple to run all tests since there are only a few hundred of them. But in the future, our capability of auto-generating billions of tests would require ML to help ensure that the right tests are being executed to deliver a robust product. Also, in the future, with billions of test cases, highly complex systems, IoT systems interacting with each other and emergent behavior, it will not be feasible for development teams anymore to manually define what correct behavior is for each test case.
So, the future of IoT testing is all about AI and ML. Intelligent testing, using both of them, is about demanding more actionable intelligence from testing: when automation, cognitive systems, and advanced analytics become integral parts of a testing ecosystem, expectations should be high, especially when proactively dealing with issues around user interfaces and user experience.
To sustain the rapid growth any IoT Testing Company is witnessing, intelligent testing is now a must. By incorporating AI and machine learning, testing is not only more intelligent, but it will become a critical business accelerator for those IoT businesses that embrace it.